Why we’re making the shift to outcomes-driven grantmaking
Posted on 12 Oct 2021
By Kathy Richardson, executive director, Our Community
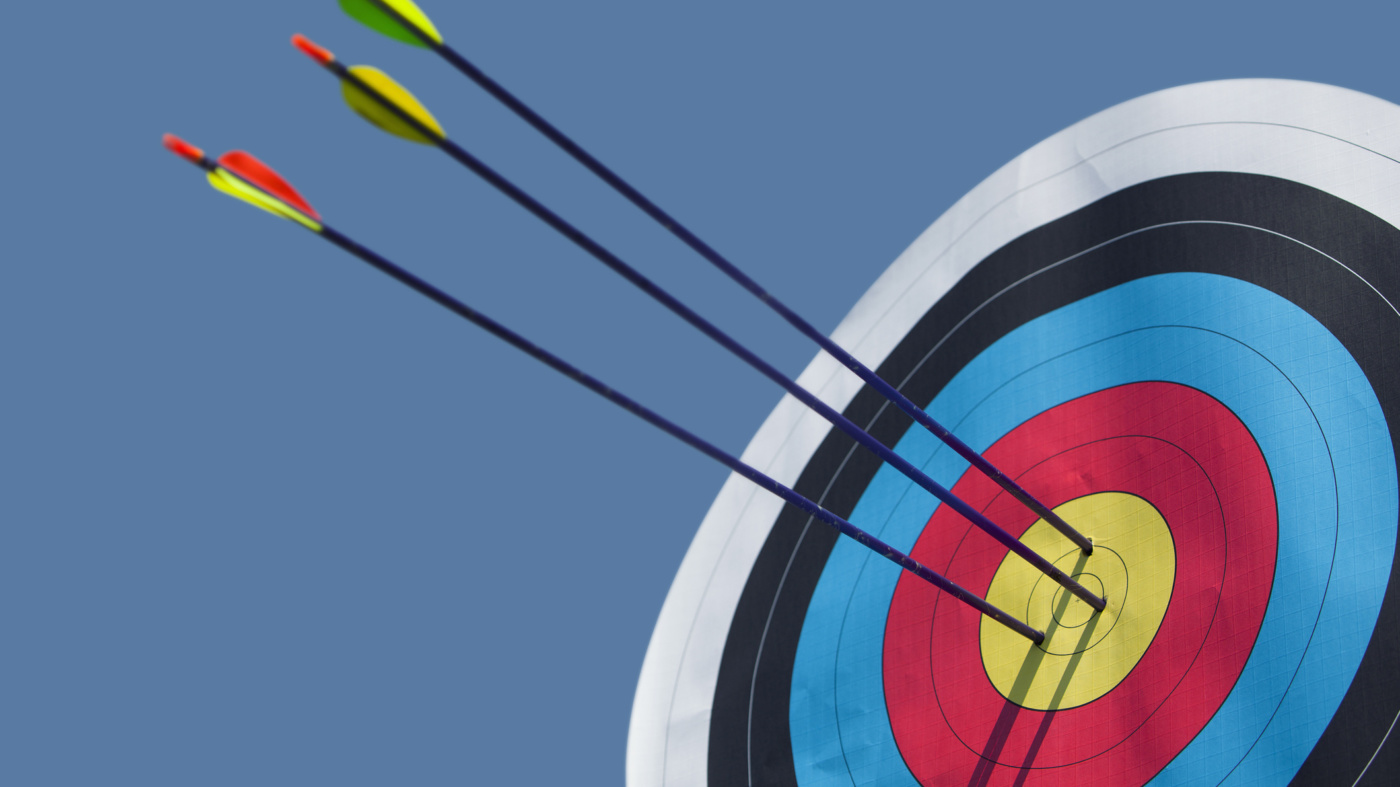
Around $80 billion is given out in grants each year in Australia, according to our best calculations at SmartyGrants. Yet in many instances no-one is quite sure what is the purpose of that funding, and no one knows what the result of that funding has been.
Our central quest at Our Community, then, is to shift all those billions of dollars from noise to meaning. We are committed to helping nudge grantmaking towards outcomes-focused practice in several ways, including through:
- education and advocacy
- promoting a learning mindset for grantmakers
- creating a shared language for social sector initiatives
- a new tool to ease the grants rage endured by not-for-profits, called SmartyFile
- an Outcomes Engine for SmartyGrants to help grantmakers with social impact measurement
As well as operating SmartyGrants, Our Community assists not-for-profits with grantseeking, fundraising, governance training and data science.
That combination of work gives us a unique vantage point from which we can view the challenges, opportunities and trends – when it comes to getting results – in both the grantmaking and not-for-profit sectors.
A large portion of those $80 billion in grants is allocated to project-based support for not-for-profit organisations.
Those not-for-profits are the engine room for social progress, while grantmakers grease the wheels with funding and provide fuel for the tank to support the outcomes they are after.
Grantmakers, you’re doing it wrong
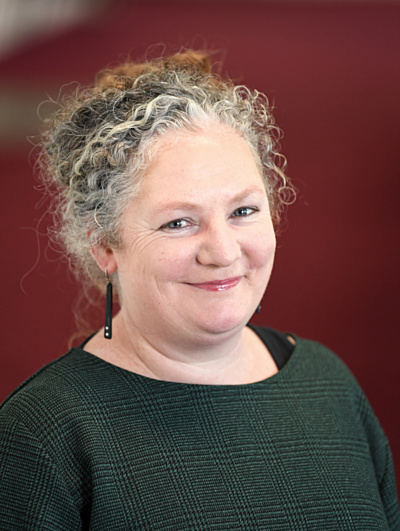
But there’s a problem. For many years, most grantmakers have been aiming at one thing but reporting on another.
While their program objectives – the grantmaker’s outcome goals – might be to build stronger communities, say, or to support the disadvantaged, to improve the environment, to enhance tourism, or to improve employment opportunities for young people, what they’ve been monitoring and reporting on is something quite different.
Mostly they’ve been concerned about:
- how much money went out the door
- to whom the money was given – what types of organisations; in what locations
- whether the money was spent appropriately, often asking for the receipts.
Recently, it’s been more common for grantmakers to ask grantees to report on:
- who have been the beneficiaries of the program that was funded
- what activities took place
- metrics such as the number of courses, the number of participants or the number of women with disability reached.
While this type of reporting shares a border with the territory of outcomes, it’s only partway there, because mostly these things are concerned with outputs.
I’m not saying that grantmakers haven’t been interested in outcomes – some have gone to a lot of trouble to work out a sophisticated set of program goals. However, barriers to good outcomes-driven grantmaking remain.
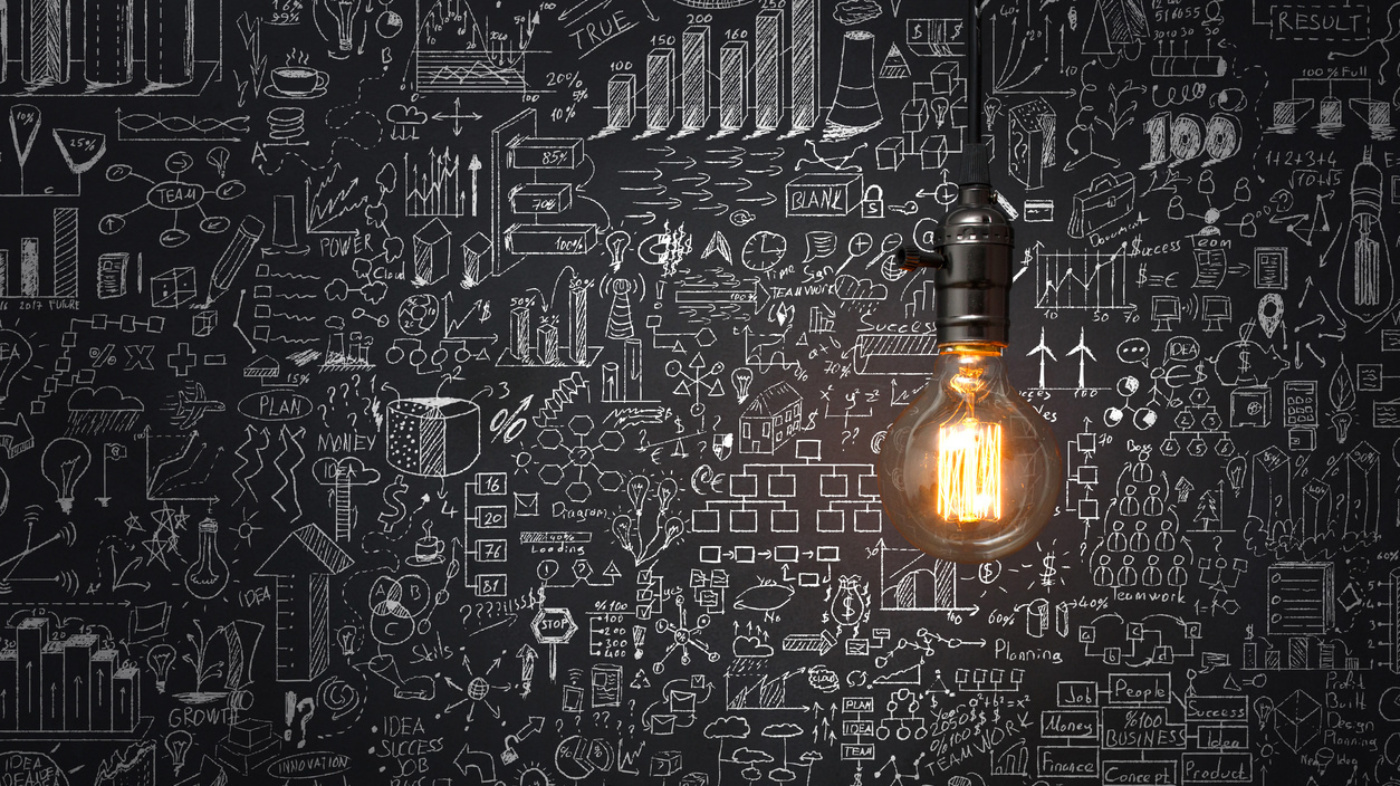
Problem #1: It’s complicated
It is hard enough to assess impact when you’re looking at a single program run by a single organisation. Aggregating across many programs and many organisations is mind-bendingly complex.
This is problem number one for grantmakers, and it’s a really big one.
I am unfortunately speaking from experience, because for the past five years or so I’ve been part of a group of half a dozen people at Our Community – tech experts, grant experts, data scientists, evaluation experts – who have been focusing really hard on this question: how can we meaningfully track and aggregate outcomes data?
Not just to allow aggregation from grant to grant for one grantmaker, but potentially to aggregate and join the dots across the whole grantmaking industry. Only then will we start to see patterns emerge that will help inform our next moves.
Job generation, a mock case study
Let’s take the example of the grantmaker who is interested in improving employment opportunities for young people. Let’s say they do some research and discover that teaching young people to drive – helping them get their licences – can help them into jobs. They release some grants to fund some learn-to-drive programs.
They might provide $100,000, say, in differently sized grants to four grantees. Then they ask the grantees to report on two things:
- How many programs did they run?
- How many people did they teach to drive?
So let’s say we have a total of 20 programs run across those four grantees, with a total of 500 people attending those 20 programs.
The grantmaker collects that data from those four grantees and pulls it together for their annual report. The minister/council/trustees/funders/donors are happy. The people who ran the courses are happy. And presumably the people who took part in the courses are happy.
But I’m still not happy. Because if I am that grantmaker or the person who funds that grantmaker (which is actually me, if it’s a government grantmaker, because my taxes pay for that grant), I want to know: how many of those kids who did the program got a job?
So let’s look at what happen when the grantmaker asks the grantees that question too. And let’s say that they had varying degrees of success.
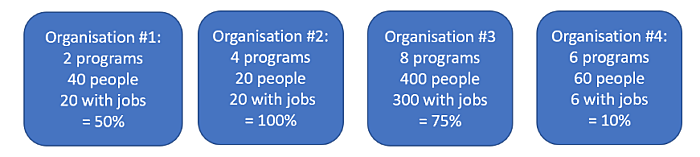
I also might want to know whether or not those job stuck. So, I might need to come back to all those grantees a year or so later to ask them how many of those kids are still in jobs.
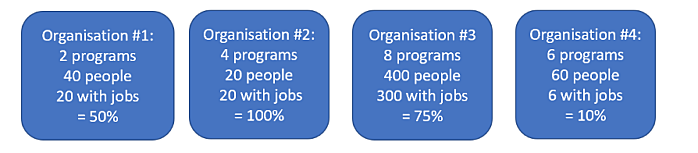
But even if you ask that, those raw figures aren’t really telling the whole story either. You need the context of the data being reported.
For example:
- What were the backgrounds and existing capacities of those young people?
- Was there a gender difference in results?
- Did organisations reporting their results all have the same definition of “doing” the course? Does turning up once for a four-week course constitute participation?
- Were the courses different in style or duration? What other differences were there?
- What, exactly, does the grantmaker mean by a “job”? Do part-time, casual or voluntary positions count?
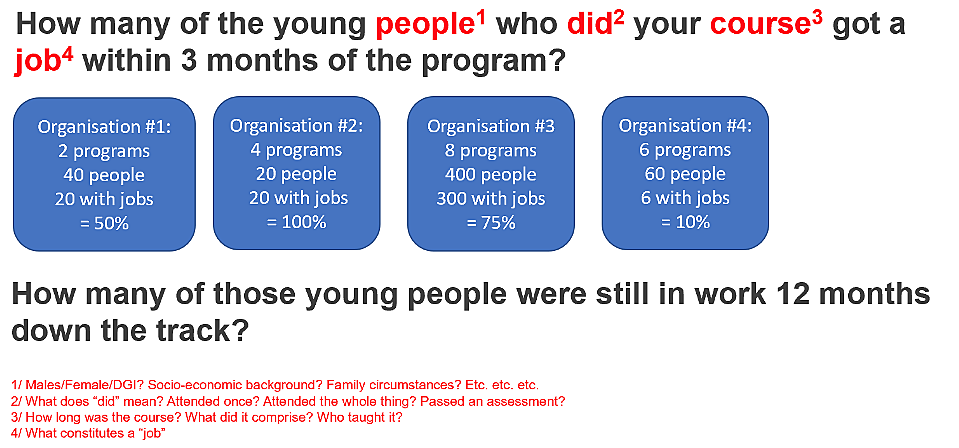
This exercise demonstrates the complexity faced by grantmakers who want to shift to outcomes-driven funding.
It’s quite a leap from “our funding supported four organisations to run 20 learn-to-drive programs involving a total of 500 people” to “40% of people who attended a learn-to-drive program went on to get a job within three months of the program, and 90% of those were still in employment 12 months later”. And beyond – “The keys to running a successful learn-to-drive program appear to be …”.
Problem #2: Grantmakers aren’t rewarded for understanding outcomes
There’s another big problem. Grantmakers are not often incentivised to collect information that will help them understand the outcomes of their funding.
They’re rewarded for getting the money out the door efficiently, preferably with some good photo opportunities thrown in. They’re rewarded for avoiding controversy and for avoiding misappropriation of funds. They’re not rewarded for changing the world. And they’re definitely not rewarded for highlighting what did not work, which in my view is absolutely just as valuable as knowing what did work.
This is why we so often end up in outputs land, surrounded by receipts.
Problem #3: Grant recipients aren’t rewarded for understanding outcomes either
Grant recipients aren’t rewarded for detecting and highlighting lessons either. As a result, they’re drawn towards gathering outputs and, where requested, showcasing winners, rather than showcasing actual results.
Those happy pictures may apply to only 20% of the participants. We don’t hear about the 75% for whom the intervention did not work at all, and definitely not the 5% who actually experienced an adverse effect. Those things are completely hidden from view. Which means we can’t learn from them.
The result of this is lots of happy pictures and lots of exaggerated outputs.
So you can see that the whole system is set up to dissuade us from an outcomes lens.
Problem #4: No one really cares about the evidence and facts
Now I hate to be endlessly negative, but here’s another problem. One conclusion I have reluctantly come to is that many, many people actually don’t care about evidence and facts.
It might shock you, but that includes governments.
A well-meaning grant officer might go to a whole lot of trouble to define outcome goals, based on sound policy and a flawless theory of change, write up some excellent guidelines, and run a competitive program that seeks to apply a merit lens to applications, only to have their spreadsheet colour-coded and changed by the people who sign the papers.
And it’s not only governments who sometimes ignore inconvenient truths. Not-for-profits sometimes ignore them as well. I’ve seen it. Some are frightened to look too carefully at whether or not their program is working. They’ve been doing it for ages; common sense tells them it should work; surely it works; we should trust our guts; it’s the journey that matters.
And it’s not always about not wanting to know. Sometimes (actually, usually) it’s just hard to find the time and money, amidst doing the work, to find out what’s truly happening. Putting in place a sensible measurement and evaluation framework can be complex and expensive.
Grantmakers too often don’t have the tools they need to “do outcomes”. They have money to give away, but not much to spend on themselves. They’re stretched, lacking capacity, and often lacking support from higher up to do these things that don’t necessarily provide a quick sugar hit.
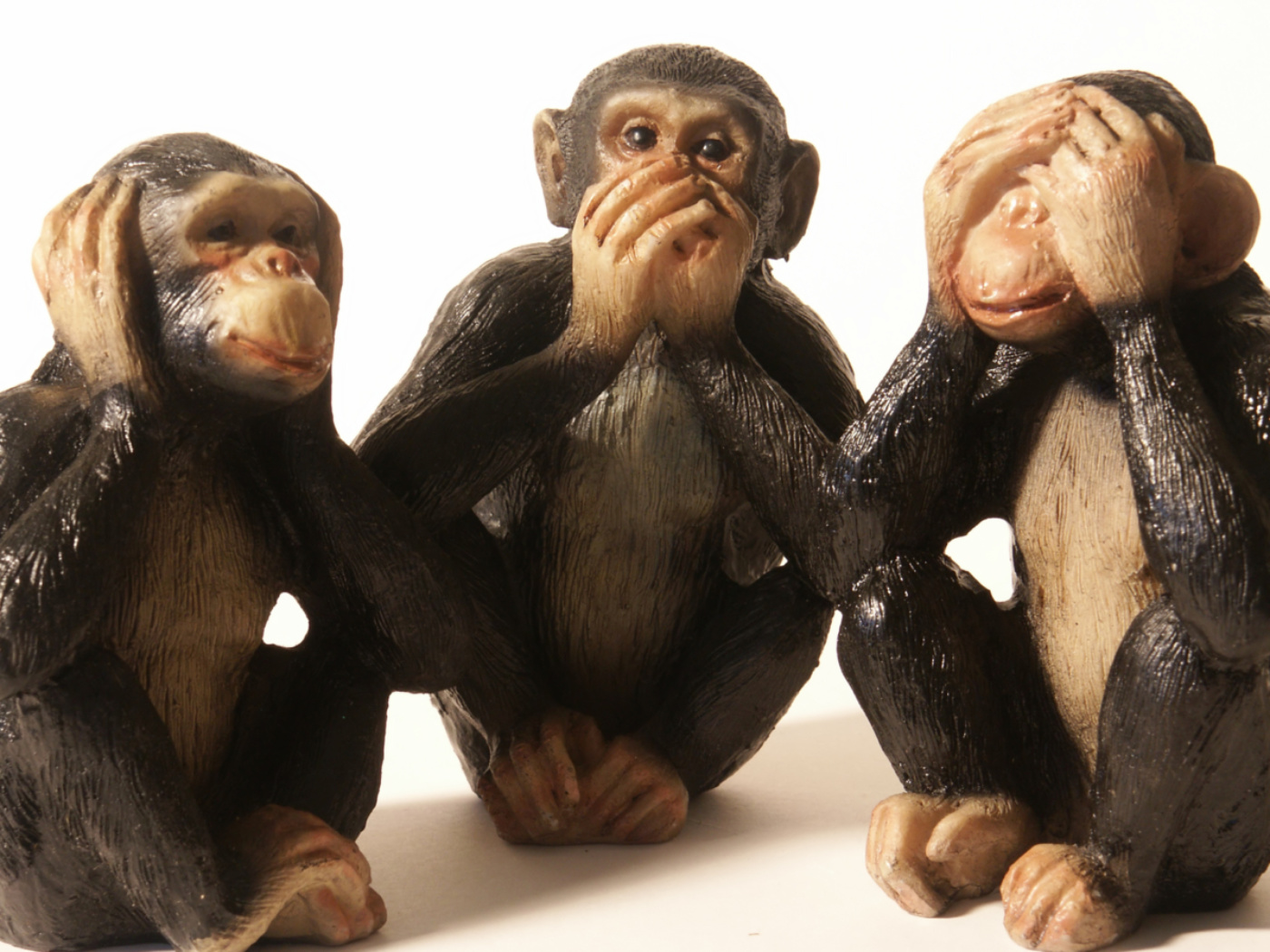
Yet more problems, for grantmakers and by them too
And then there are the difficulties that apply to anyone wanting to track outcomes. Here are some of them:
- Attribution is tricky.
- Every answer throws up another question.
- Some evaluation processes can produce misleading results, which can take ages to get right.
- The attention span of funders’ managers can be fickle.
- Grantmakers rely on not-for-profits to provide them with the data they need, but they don’t want to fund them to collect it.
- Grantmakers want not-for-profits to innovate, even if they’re doing things that are working.
In fact, many grantmakers have got into some pretty bad habits when it comes to working with not-for-profits. Not-for-profits are often punished if they spend too much money (or, in many cases, any money at all) on salaries, overheads and administration, although nobody can explain exactly how they’re supposed to distribute surveys without paying for a surveying tool and employing an analyst to make sense of the results.
Not-for-profits are looked down upon – perhaps even put on the blacklist – if a funded project turns out differently from what was expected. And as I said before, knowing what doesn’t work – and why – is just as important as knowing what does work.
All of this results in a system that’s about outputs, that’s about penny-pinching, and that’s about reporting, not learning. It’s about talking up the positives rather than reflecting on how to do better next time; it’s about avoiding complexity (when complexity is where all of the good stuff actually lives) and obsessing over headcounts. The system has in-built design faults and they are working against those of us wanting to shift to evidence-based funding.
How SmartyGrants is trying to solve the outcomes puzzle for grantmakers
So what’s to be done?
I can’t claim that we’ve solved all of the problems. But we’re also not letting knowledge of the problems distract us from our central quest – that is, as I said earlier, helping to shift those $80 billion from noise to meaning.
Mostly we are doing that using our software system SmartyGrants, which grantmakers use to manage grants from application to acquittal (our “benevolent Trojan horse”). The system was launched in 2009 and has grown quickly, now being used by 450-odd organisations to dispense billions each year.
But SmartyGrants is more than a software solution. Before we built SmartyGrants we tracked best practice grantmaking around the world as the Australian Institute of Grants Management and distributed that knowledge to grantmakers through our website, newsletters, whitepapers, conferences and networking events.
We have been doing that for about 20 years now and we have embedded those lessons into the software and shared it with our community of grantmakers.
All of them use SmartyGrants to create efficiencies, but many also share our dream of becoming more effective.
Promoting a “learning mindset” for better results
In the past we have imagined the grantmaking process as linear. You start with a policy problem, mount a grant program (as one of the ways to get to some solutions), collect applications, give the grant, they do the project, you get the final report convincing you the money was well spent and not misappropriated, and you’re done.
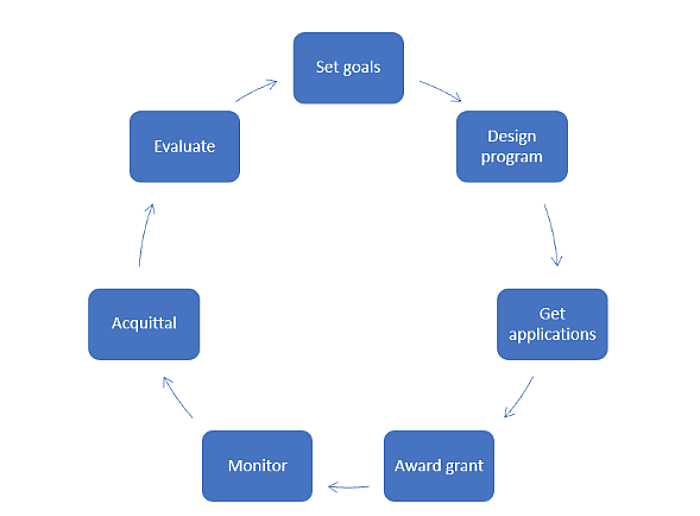
Of course – and this seems obvious now – it’s not linear at all. It’s circular. Or it should be.
At the end of each grant (indeed throughout the process), there must be an analysis of outcomes, and then you have to use that knowledge to inform the next round, and the next. If you don’t, you’re missing out on a big opportunity for improvement, which means you’re wasting a lot of precious money.
At high school, in science classes, we were taught to identify a problem, do some research, pose a hypothesis then set about testing it. We didn’t get marked down if our hypothesis was not supported by the results. The experiment was only a dud if we failed to learn from it, or if we failed to share what we were learning with others, if we failed to progress. That’s how it should be with social change programs. We have to learn what works a little at a time.
That also means changing how grantmakers interact with grantees.
We have some tools built into SmartyGrants to encourage this behaviour.
Our template forms and inbuilt standard fields include questions like “What’s the need and how will you address it?” and “How will you know if it’s worked?”
Our acquittal forms don’t just ask about expenditure, they ask, “What did you learn as a result of undertaking this project?”
Now, SmartyGrants users can build their own forms – they can ignore us entirely – but if they use our template forms as a basis, or they use our standard fields, they’ll have a learning slant to their programs.
Creating a shared language
Another thing we realised we needed to do was to get everyone speaking the same language, because there’s not much point in everyone learning in isolation.
You may have heard of our taxonomy project – CLASSIE (Classification of Social Sector Initiatives and Entities). CLASSIE is like a dictionary for the social sector. It helps us categorise:
- organisations – what type of not-for-profit or grantmaker, how big you are, how much income, how many staff, etc.
- subjects – what sort of work you do (arts, environment, public health, employment, etc.)
- populations – most commonly it’s used to identify the beneficiaries of a project (people with disabilities, migrants, homeless people, women, animals, farmers etc.).
Recently we took this one step further. Our data scientists have developed an algorithm dubbed CLASSIEfier that will effectively “read” a grant application and apply subject and beneficiary labels automatically.
In fact, it can read any piece of text and classify it according to subject and population; it can also classify according to the UN’s Sustainable Development Goals. CLASSIEfier has just been released into SmartyGrants.
The point of this is to reach a state of shared learning where we are all labelling the same things in the same way, enabling us to find similarities in a sea of data. That’s the only way we can compare and contrast and learn what works.
CLASSIE has been available in SmartyGrants for a couple of years and it’s now being used more widely, including by the Australian Charities and Not-for-profits Commission (ACNC). We regularly receive and review user feedback, and updates are released every six to 12 months.
Outcomes Engine: creating clarity for grantmakers
Our most exciting project, for those measuring outcomes, is the aptly named Outcomes Engine. Following five years of development, it now being used by 18 pioneering grantmakers.
The Outcomes Engine works with all the tools grantmakers (and hopefully grantees) are familiar with, including form building, standard fields and reporting.
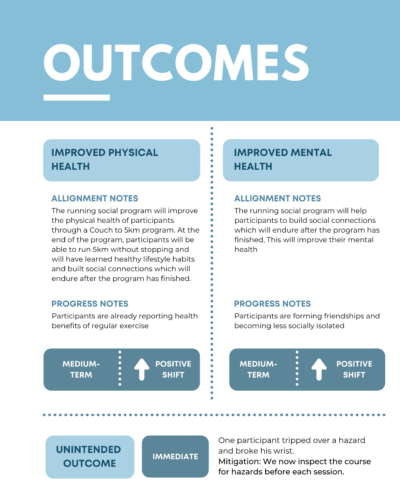
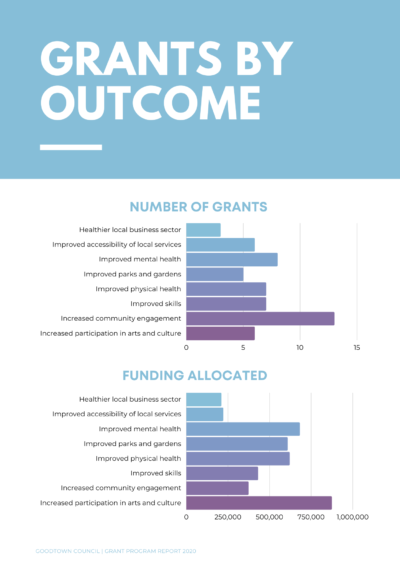
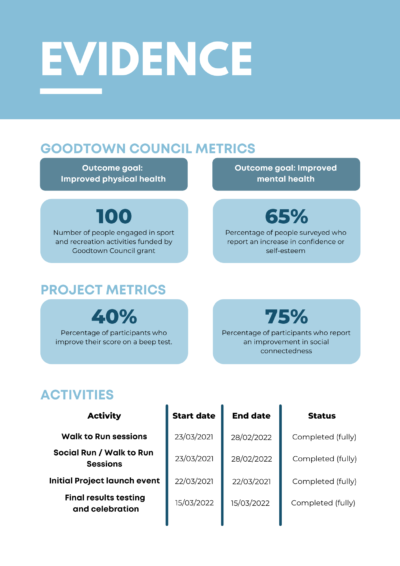
The system is mostly self-serve for grantmakers and consists of three main parts:
- the Outcomes Framework (the grantmaker’s) which can be as simple as a couple of outcome goals; or it can have many, many entries
- standard sections – a group of standard questions that you can put on a form, or on multiple forms, to build up a picture over time
- reports.
Grantmakers upload their selected outcomes framework into their system, with their chosen domains, outcomes and metrics. There are common frameworks available if they don’t have one of their own, or they can create multiple frameworks within the same account.
Some questions for grantees will appear on applications, such as “What metrics will you use to track your progress?”, while others will display on progress or final reports, such as “What is your progress to date?”
These questions allow the grantee and grantmaker to create a picture of progress over time.
Importantly, we encourage grantmakers to ask grantees about their own outcome goals and key performance indicators as well, rather than just imposing their own on their grantees.
Each section comes with some introductory text (“What is an outcome?” What do we mean by a metric?”) with some suggested wording, hints and validation rules.
Grantmakers can choose questions that are relevant to the work and capacity of their grantees, as well as add their own questions, change the question order, and set their own validations.
The idea is to help them create or embed their own logic model into their grantmaking, and tap into their grantees’ logic models as well, and to get readable, meaningful reports into the hands of grantmakers and grantees.
Aggregate reports can be generated to show outcomes, metrics and activities grouped by domain or outcome.
Importantly, we want to make sure that the data is not available only at the end of it all. Our reporting system allows grantmakers to view and use outcomes data at any point in the process – including during assessment of applications, and then in progress reports as well.
In time, we hope, information will flow directly, without the need for formal reporting at all, from the grantee’s record-keeping systems, into the grantmaker’s.
Our hope is that as more and more grantmakers start using these fields on their forms we can help them learn more about their impact. What we’re trying to do is to guide them in setting up a data schema that will help them answer that question: “What needle(s) did our money help to shift?”
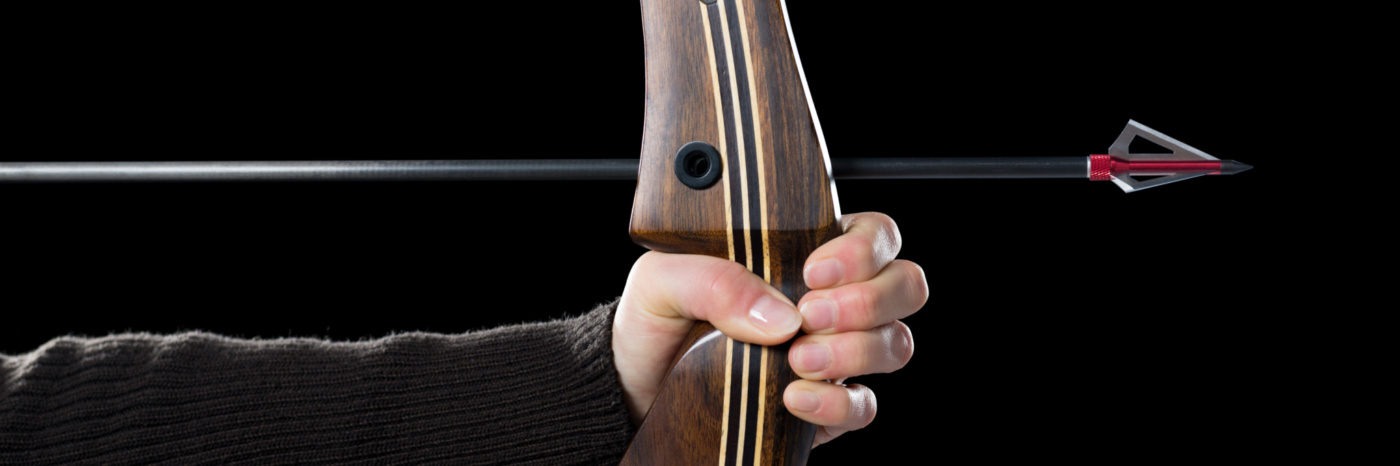
The future: The Centre for What Works
Really good grantmakers want to know what worked, what didn’t work, why, and how they can do better next time. But why would they want to be able to learn only from the programs they themselves funded?
Come to that, why would the funded organisations not want to benefit from the wisdom of the crowd?
Let’s return to that learn-to-drive program. It’s all well and good to know that 80% of participants in 20 programs went on to get jobs, but I also want to know if there were some programs that were more successful than others. And if there were, what made them better?
When I open my next round of learn-to-drive funding, I want to know which programs I should be funding, and which organisations I should be funding.
If I’m a not-for-profit organisation, I want to be able to get a quick rundown of what works and what doesn’t when you’re running a learn-to-drive program.
For years now, pretty much as long as I have been at Our Community (about 20 years), we have had a plan to create this better picture of what works to create social change.
If you look on the Our Community website, you’ll find a section called The Centre for What Works. It’s been up there for more than 15 years, without there being very much to put in it.
Well, soon we hope to be able to populate that site. Once we have enough data flowing through those fields in SmartyGrants, we should be able to see patterns and opportunities for shared understandings. We can start comparing apples with apples. And because the data is categorised properly, we can find all the pieces of context we need, when we need them.
What we want to be able to do is to drag the lessons out of the Centre for What Works and into SmartyGrants: serve them up to grantmakers, and to grant applicants as well, at the most useful time for them.
There’s lots of good evidence being produced already, but not much of it is being read, or used to inform future practice. We’re hoping to be able to create the demand by serving up the right piece of data, to the right person, in the right format, at the right time.
With permission, of course.
That’s the point of the whole thing – so we can all push forward together.
More information
SmartyGrants founder spells out the agenda for a mature sector
More about the Outcomes Engine
Six hot tips on using SmartyGrants to track grantmaking outcomes
Top bureaucrat: Why it's so hard to move from outputs to outcomes
Expert advice on reporting: progress reports, acquittals and outcomes